Bringing 3D gait analysis technology to clinical and community settings
The technology serves as a digital biomarker of disease progression, recovery and rehabilitation outcomes
Markerless motion capture (MLMC) technology uses complex neural network algorithms to analyze 3D body motion. It provides detailed analysis that can help identify potential neurological conditions, such as Parkinson’s disease, and monitor disease progression across many health conditions.
In a recent study published in Frontiers in Human Neuroscience, UC Davis Health researchers tested the feasibility of using MLMC in community settings. They were particularly interested in determining whether it can be used as an outcome measure to track the progress of rehabilitation for neurological conditions.
Their study found that MLMC had great potential in both clinical and community settings and with a diverse population.
“We found that this technology can be used as a 3D digital biomarker to detect how neurological conditions affect peoples’ movement,” said senior author Carolynn Patten. Patten is the director of the Biomechanics, Rehabilitation, and Integrative Neuroscience (BRaIN) Lab and a professor at the Department of Physical Medicine and Rehabilitation.
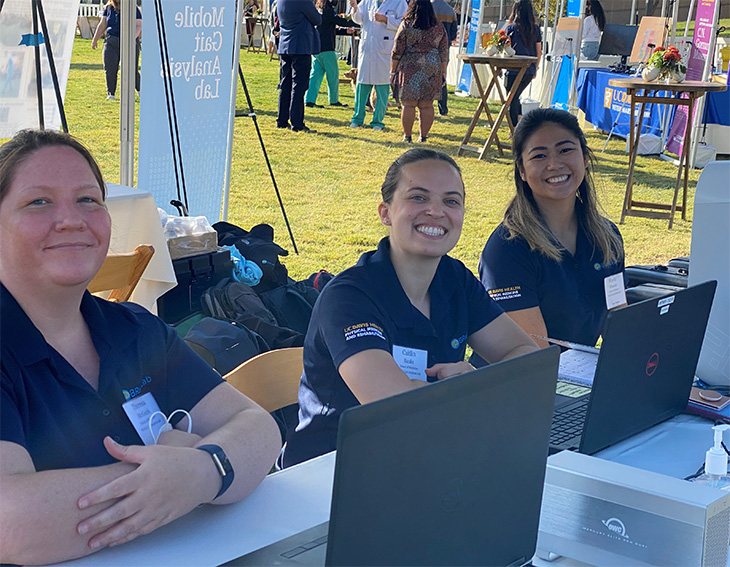
Taking the technology to the community
The research team wanted to assess gait in an everyday environment that was more natural and accessible.
“We took this 3D MLMC technology and ventured outside the dedicated motion capture lab into the ‘real world’ of our local community,” Patten explained.
The team recruited 166 participants from six community locations, including a sports field and a lobby adjacent to a health clinic. They measured the participants’ height, weight and leg length and gathered their health history and demographics. Unlike studies conducted in the traditional motion analysis lab, the participants wore their own clothing and footwear.
We ventured outside the dedicated motion capture lab and took this 3D MLMC technology into the ‘real world’ of our local community.” —Carolynn Patten
“One of the advantages of MLMC is the ability to study people without the need to place reflective markers on body landmarks or the need for specific clothing,” Patten commented.
The team used eight video cameras to capture data from participants performing two tasks: first, to walk as they usually do, then to walk as fast as possible. They measured 12 parameters, including cadence, speed, step length, stride length, stride width, step time, and stride time.
“The entire process from when we first met a participant and they agreed to take part until we were finished collecting data took less than half an hour. In the traditional lab, the same experiment requires at least 1.5 hours after arrival,” Patten said.
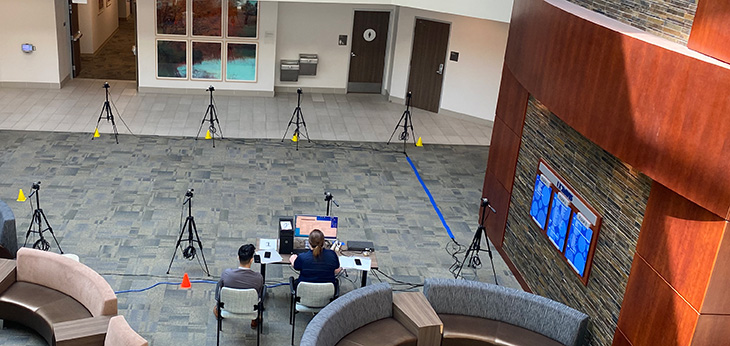
The findings: Accurate spatiotemporal measurements
The participants’ ages ranged from 9–87 years. They had varied health histories, but all could walk at least 15 meters independently, even if using an assistive device or brace. A subset of 46 participants walked over a pressure-sensitive walkway concurrently with MLMC. This allowed researchers to compare the parameters and assess agreement between the two systems.
The study found that the measures reported by both systems were quite similar. Values for cadence, speed, step time, stride time and stride length appeared identical.
It also showed that it is possible to acquire high-resolution 3D motion data outside the traditional laboratory, in clinical as well as non-clinical settings.
“The technology itself can be brought to the patient and clinician, rather than vice versa,” Patten noted.
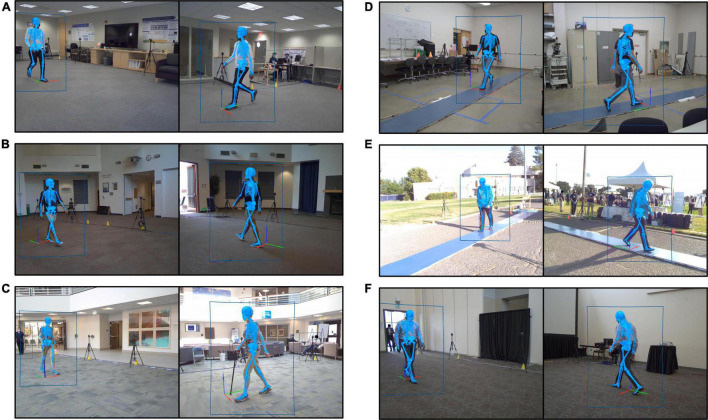
Developing rehabilitation strategies
According to the researchers, access to MLMC information can offer many advantages for providers, including those managing cases of complex neuromotor dysfunction. It replaces the need to rely on observations and approximations to understand motor dysfunction.
More critically, this high-resolution 3D information can augment the clinician’s assessment. It sets the stage for data-informed clinical practice and the development of customized rehabilitation strategies based on the individual’s specific movement deviations.
“Our purpose is to consider current and evolving tools and how they can enhance understanding and better inform neurorehabilitation,” Patten said. “Whether the intervention targets strengthening strategies, pharmacologic agents, or biologic interventions, such as stem cell infusions, 3D gait analysis provides a robust and sensitive digital biomarker.”
The study’s lead author is Theresa McGuirk. The co-authors are Elliott Perry, Wandasun Sihanath, and Sherveen Riazati. McGuirk, Perry, and Patten are at UC Davis Department of Physical Medicine and Rehabilitation and affiliated with the Veterans Affairs Northern California Health Care System.
This work was supported by the UC Davis Healthy Aging in a Digital World Initiative and the Tsakopoulos Demos Aging and Healthy Communities Fund. Additional support was provided by the UC Davis School of Medicine and the VA Rehabilitation RR&D Service through a Research Career Scientist Award (#IK6RX003543). The UC Davis Clinical and Translational Science Center (CTSC) also gave the researchers access to REDCap. REDCap is a tool supported by the National Center for Advancing Translational Sciences (NCATS) and National Institutes of Health (NIH), through grant UL1 TR000002.